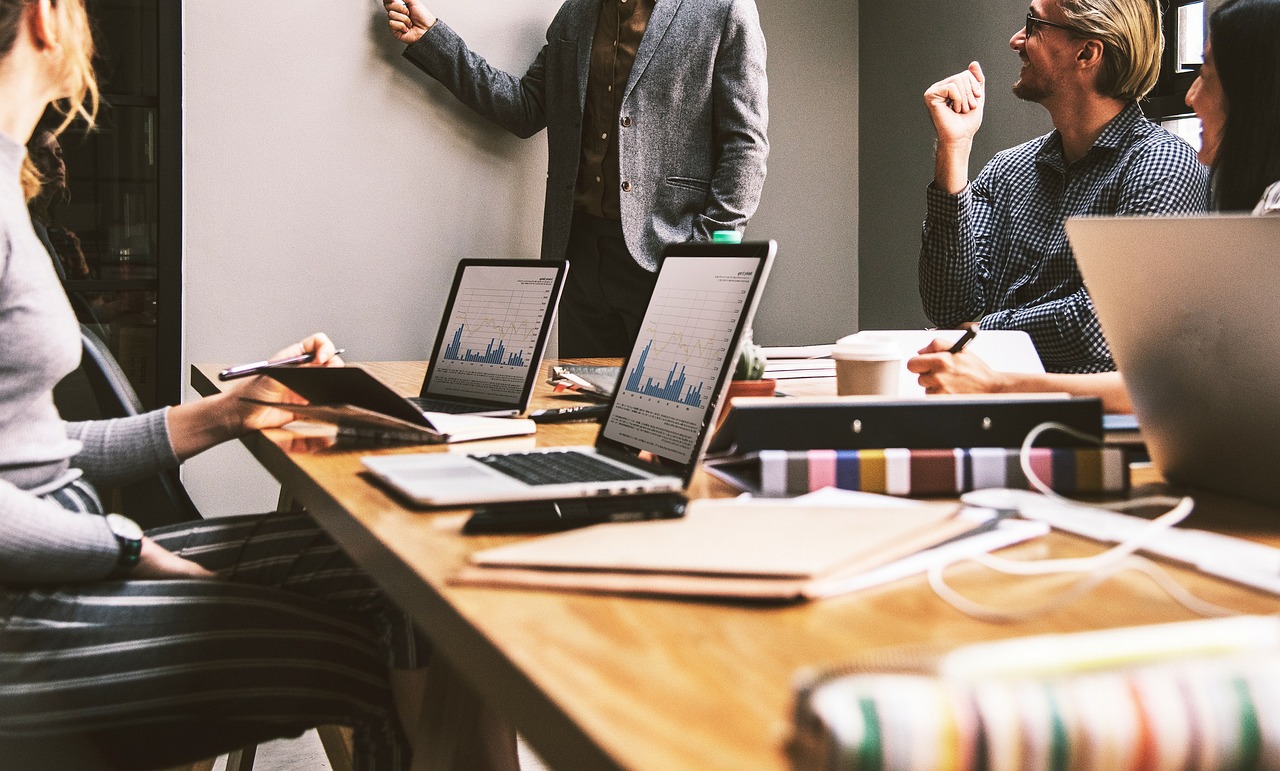

Advisory & AI Consulting
We partner with organizations to drive AI-enabled transformation, combining strategic insight with deep technical expertise. From platform architecture to custom model development, we help teams unlock the full value of their data and technology investments.
Core Domains
AI & Data Strategy
Custom AI development
AI & Data Strategy backed by Experience
We bring deep, hands-on expertise to building, assessing, and scaling AI and data systems. Our work combines strategic insight with technical precision, rooted in real-world experience. We have ourselves engineered platforms, refined architectures, and delivered impact in the same environments our clients face today. We support our clients to:
Identify high-impact use cases and align them with business value.
Guide adoption of LLMs, GenAI, and agentic AI based on real product use—not trends.
Assess existing architecture, tooling, and teams to uncover gaps and risks.
Shape pragmatic AI roadmaps—prioritized, budget-aligned, and technically feasible.
Recommend technologies, vendors, and team structures to support long-term scale.
Build the first 100-day implementation plan to operationalize AI from day one.
We propose in-depth Technical Assesment, whereas for internal evaluation or the purpose of due dilligence for M&A or investments, including
Architechture and infrastructure assessment
AI/ML capability and team maturity evaluation
Data pipeline, tooling and security stack analysis